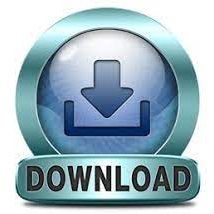
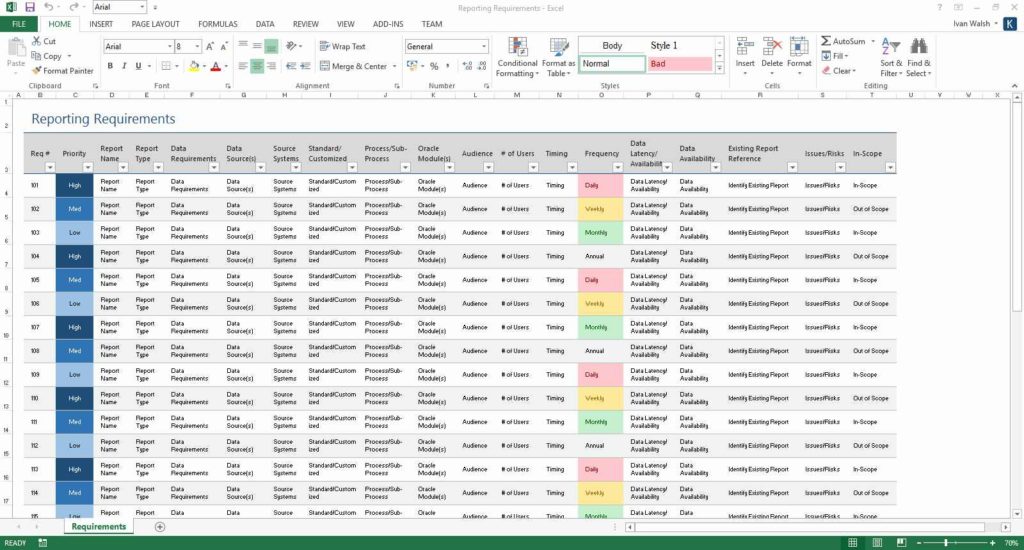
Having set the goal of reducing dimension of our data set to a smaller number of factors a simple choice would be to use the average. This is often called a reduction in the data set’s dimension. With so many variables it may be easier to consider a smaller number of combinations of this original data rather than consider the full data set. Our data set has nine variables in total.
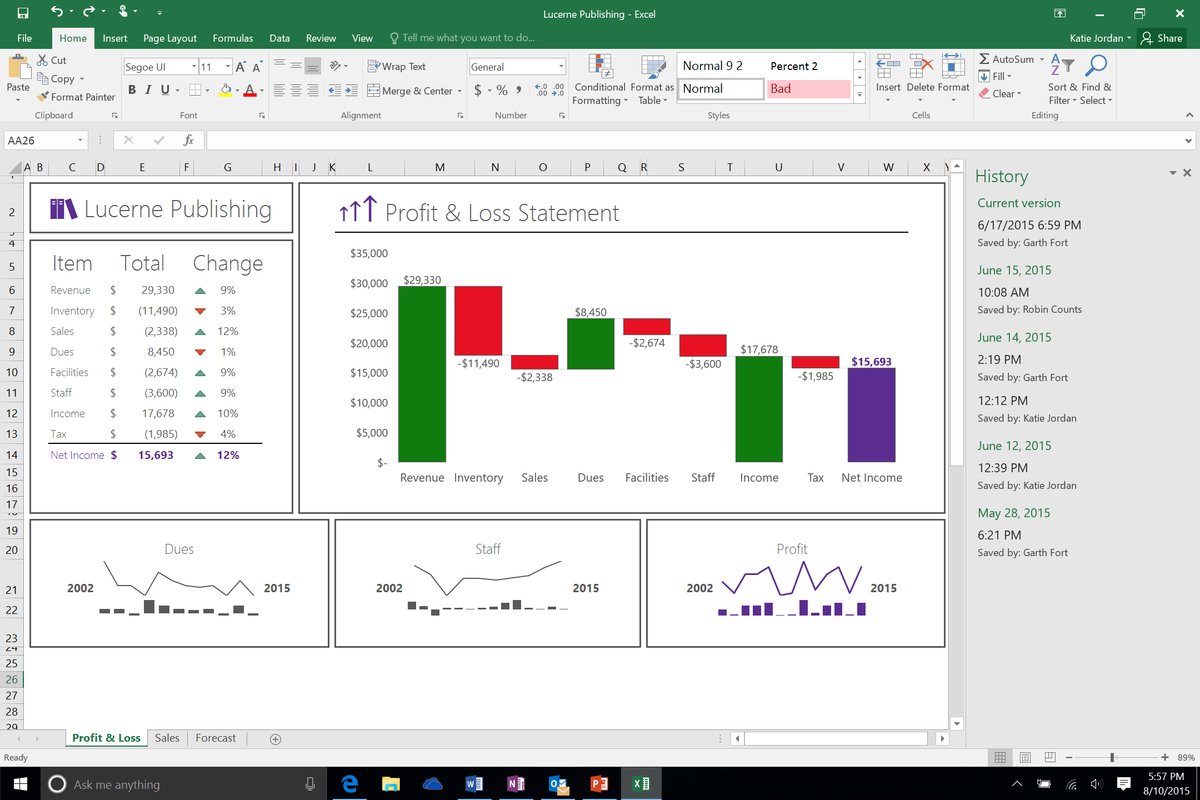
We consider changes in 2y, 3y, 4y, 5y, 7y, 10y, 15y, 20y, 30y swap tenors. For example, we may have a time series of daily changes in interest rate swap rates for the past year. The idea of PCA is to find a set of linear combinations of variables that describe most of the variation in the entire data set. Alternatively the reader can download this excellent addin for free from. This book comes with a free excel addin Matrix.xla that can be used to implement PCA in excel. One book which we really like is Carol Alexander’s Market Risk Analysis Volume 1. For those who are interested to know the mathematics behind this technique we recommend any multivariate statics book. In practice it is less important to know the computations behind PCA than it is to understand the intuition behind the results.

In the current post we give a brief explanation of the technique and its implementation in excel. We decided to write a series of posts on a very useful statistical technique called Principal Component Analysis (PCA).
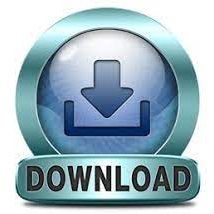